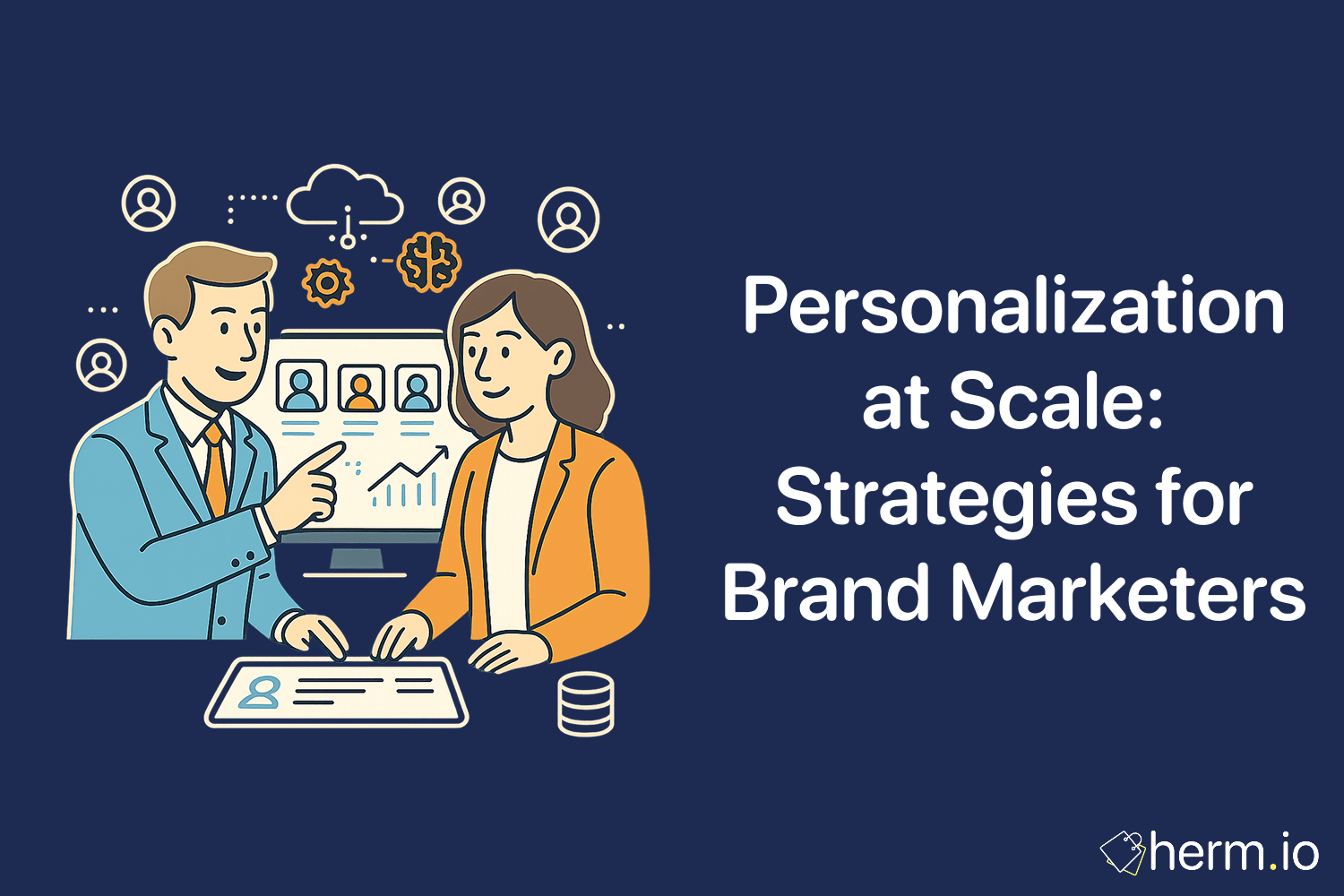
Imagine a world where every customer interaction with your brand feels as personally tailored as a conversation with an old friend. In today's digital landscape, this level of connection is not only possible but increasingly expected by consumers who navigate a sea of competing messages daily. Personalisation at scale, once considered the holy grail of marketing, has evolved from aspirational concept to operational necessity for brands seeking meaningful engagement in an age of information abundance.
This comprehensive exploration delves into the art and science of delivering individualised experiences to vast audiences without sacrificing the human touch that makes such connections valuable. You will discover practical frameworks for implementation, examine real-world success stories, and gain actionable insights to elevate your brand's personalisation strategy beyond the commonplace.
The Essence of Scalable Personalisation
Defining Personalisation at Scale
Personalisation at scale represents the sophisticated orchestration of data, technology and human creativity to craft experiences that resonate with individual customers across your entire audience spectrum. Unlike traditional mass marketing approaches that broadcast uniform messages, advanced personalisation harnesses rich customer insights to deliver communications that speak directly to specific needs, preferences and behaviours.
Consider the skilled sommelier who remembers not just your name but your palate preferences, recommending the perfect wine from thousands of bottles based on your past selections and subtle reactions. Similarly, brands that excel at scalable personalisation analyse behavioural patterns and preference signals to present customers with the most relevant content, products and experiences at precisely the right moment.
The technical foundation involves intricate systems that process substantial quantities of customer data to identify meaningful patterns, then automatically generate tailored interactions across multiple touchpoints. However, truly effective personalisation transcends mere algorithmic recommendation; it infuses these automated processes with brand values and creative intelligence that maintain a distinctly human quality regardless of scale.
The Strategic Imperative
In a marketplace where attention has become the scarcest resource, personalisation serves as the critical bridge connecting data-informed decisions with emotional brand resonance. Contemporary consumers, confronted with an estimated 5,000 brand messages daily, instinctively filter out generic communications while gravitating toward experiences that demonstrate understanding of their individual context.
Research consistently validates this strategic importance: McKinsey analysis reveals that companies excelling in personalisation generate 40% more revenue than average counterparts. Similarly, Epsilon research indicates that 80% of consumers are significantly more likely to purchase from brands offering personalised experiences.
The competitive advantage extends beyond immediate conversion metrics. Properly executed personalisation establishes a virtuous cycle where each interaction enriches your understanding of the customer, enabling progressively more relevant communications that foster deeper loyalty and advocacy. This compounding effect transforms traditional customer relationships into dynamic, evolving partnerships that become increasingly difficult for competitors to disrupt.
Data-Driven Foundations
The Analytics Ecosystem
Robust marketing analytics serve as the navigational system for personalisation efforts, providing the essential insights that transform raw data into actionable customer understanding. This ecosystem comprises interconnected tools and methodologies that collectively reveal the multidimensional portrait of your audience required for meaningful personalisation.
A comprehensive analytics framework should integrate multiple data streams: website interactions, purchase history, email engagement, social media behaviour, customer service communications and offline touchpoints. The synthesis of these diverse signals creates a holistic view significantly more valuable than any single data source. For instance, understanding that a customer frequently browses premium products but purchases only during promotions reveals price sensitivity that transaction data alone might miss.
When evaluating your analytics capabilities, consider whether your current setup enables you to answer increasingly sophisticated questions about customer motivation and context rather than merely tracking activities. The difference lies in progressing from "what happened" (descriptive analytics) through "why it happened" (diagnostic analytics) to "what will happen" (predictive analytics) and ultimately "how can we make it happen" (prescriptive analytics).
Essential Technology Enablers
The technological infrastructure supporting personalisation at scale has evolved dramatically, making capabilities once reserved for digital giants accessible to organisations of all sizes. These tools fall into several interconnected categories, each addressing specific aspects of the personalisation challenge.
Customer Data Platforms
Customer Data Platforms (CDPs) serve as the centralised nervous system of personalisation efforts, unifying fragmented customer data into coherent, actionable profiles. Unlike traditional CRM systems focused primarily on transaction records, modern CDPs integrate behavioural signals, preference data and contextual information from disparate sources whilst resolving identity across devices and channels.
This unified customer view allows marketers to move beyond simplistic segmentation towards truly individualised engagement. For example, luxury fashion retailer Matches Fashion implemented a CDP that synthesised online browsing behaviour with in-store purchases and stylist interactions, enabling them to create consistent experiences across channels that acknowledged the full relationship history with each customer.
Artificial Intelligence Applications
Artificial intelligence and machine learning technologies have transformed personalisation from labour-intensive manual processes to sophisticated systems capable of continuous optimisation at unprecedented scale. These technologies excel in identifying patterns too complex for human analysis and automatically applying these insights to millions of individual interactions.
AI applications in personalisation typically address four key functions:
- Pattern recognition: Identifying meaningful correlations between customer attributes, behaviours and preferences that inform segmentation and targeting
- Content optimisation: Determining which creative elements, messaging approaches and offers will resonate with specific individuals
- Timing optimisation: Identifying ideal moments for engagement based on historical response patterns and current contextual signals
- Channel orchestration: Determining the optimal sequence and combination of touchpoints for each customer journey
Significantly, the most effective AI implementations maintain human oversight, with marketers establishing parameters and creative guidelines while algorithms handle execution and optimisation. This symbiotic relationship combines algorithmic efficiency with human creativity and judgement, a balance exemplified by Spotify's approach to their personalised playlists, where human curators establish quality standards while algorithms scale the personalisation process.
Implementation Excellence
Strategic Framework Development
Developing a comprehensive strategic framework provides the essential structure for personalisation initiatives, transforming abstract concepts into operational reality. This framework should address four foundational elements: objectives, data architecture, technology integration and governance principles.
Begin by establishing clear, measurable objectives aligned with broader business goals. Rather than pursuing personalisation as an end in itself, articulate specific outcomes such as increased customer lifetime value, improved retention rates or enhanced cross-selling effectiveness. These objectives should include precise metrics and timeframes, providing concrete benchmarks against which to measure progress.
Next, develop a data architecture that supports your personalisation ambitions without creating unnecessary complexity. This involves mapping existing data sources, identifying critical gaps and establishing protocols for data collection, integration and maintenance. Consider both technical and ethical dimensions, particularly regarding privacy compliance and customer consent management.
Technology integration represents the third framework component, addressing how various platforms and tools will work together to deliver seamless personalisation. This typically includes customer data platforms, content management systems, marketing automation tools and analytics solutions. Document specific integration requirements, data flows and decision authority across these interconnected systems.
Finally, establish governance principles that balance personalisation effectiveness with brand consistency and compliance considerations. This includes defining roles and responsibilities, establishing approval processes for automated communications and creating guidelines for when human intervention is required. Well-structured governance ensures personalisation efforts remain aligned with brand values and regulatory requirements whilst maintaining operational efficiency.
Practical Implementation Steps
Translating strategic frameworks into operational reality requires a methodical, phased approach. Below, we outline a practical implementation roadmap that balances ambition with pragmatism.
1. Customer Understanding Development
Begin with a comprehensive assessment of your current customer understanding, identifying both strengths and critical gaps. Combine quantitative analysis of existing data with qualitative research methods such as interviews, surveys and observational studies to develop multidimensional customer profiles.
Focus particularly on identifying the underlying motivations and contextual factors that influence customer behaviour rather than merely cataloguing demographic attributes or transaction history. For example, understanding whether a customer's purchase is motivated by personal need, gift-giving or professional requirements provides essential context for subsequent personalisation efforts.
Document these insights in detailed persona profiles that capture not just who your customers are but why they engage with your brand, what they value most and how their needs evolve throughout their relationship with you. These profiles serve as the foundation for all subsequent personalisation initiatives.
2. Content Strategy Alignment
Personalisation effectiveness ultimately depends on having appropriately varied content to serve different customer segments and individual needs. Audit your existing content assets, evaluating both quantity and adaptability for personalisation purposes. Identify critical gaps in your content library that limit personalisation potential and develop a systematic plan to address these deficiencies.
Develop a modular content architecture that enables dynamic assembly of personalised communications from standardised components. This approach balances personalisation depth with operational efficiency, allowing you to create exponentially more combinations from a manageable library of content elements.
For instance, fashion retailer ASOS developed a modular email system combining dynamically selected product recommendations, editorial content and promotional offers based on customer preferences and purchase history. This approach enabled them to generate thousands of unique email combinations while maintaining strict control over brand presentation and messaging hierarchy.
3. Implementation and Optimisation
Begin implementation with focused pilot programmes targeting specific customer segments and journey stages where personalisation can deliver immediate value. These initial efforts serve multiple purposes: demonstrating business impact, refining technical approaches and building organisational capabilities.
Prioritise use cases with clear commercial potential and reasonable technical complexity. For example, many organisations begin with personalised product recommendations or targeted promotional offers before advancing to more sophisticated applications like individualised content journeys or predictive customer service interventions.
Establish disciplined testing protocols for continuous optimisation, employing both A/B testing for tactical improvements and longer-term controlled experiments to assess strategic impact. Document learnings systematically and create feedback loops that inform ongoing refinement of both technical implementation and strategic direction.
Case Studies in Excellence
E-Commerce: ASOS's Product Discovery Revolution
British online fashion retailer ASOS faced a significant challenge: helping customers discover relevant products from a catalogue spanning over 85,000 items. Their solution was a sophisticated personalisation engine that transformed product discovery across their digital properties.
ASOS implemented a comprehensive approach combining collaborative filtering (identifying products purchased by similar customers), content-based recommendations (suggesting items with similar attributes to those previously viewed) and contextual relevance (adjusting recommendations based on seasonality, availability and promotional strategy).
The implementation involved developing custom algorithms that considered both explicit preferences (stated size and style preferences) and implicit signals (browsing behaviour, purchase history and engagement patterns). This approach enabled ASOS to personalise multiple touchpoints, from homepage content and category pages to email recommendations and retargeting advertisements.
According to their 2019 annual report, this personalisation initiative delivered remarkable results: a 30% increase in conversion rate for personalised touchpoints and a 16% increase in average order value for engaged customers. Implementation required significant investment in both technology infrastructure and data science capabilities, with a dedicated team of analysts responsible for continuous optimisation.
Financial Services: Monzo's Contextual Banking Experience
Digital bank Monzo revolutionised personal finance management through hyper-contextual personalisation that transforms raw transaction data into meaningful insights and guidance. Unlike traditional banking interfaces that present identical dashboards to all users, Monzo's app experience dynamically adapts based on individual financial behaviours, goals and circumstances.
Their approach begins with sophisticated categorisation and pattern recognition algorithms that automatically classify transactions and identify recurring payments, discretionary spending and saving opportunities. These insights power personalised notifications and guidance that evolve with the customer's financial journey.
For example, customers who regularly spend on restaurants receive customised budgeting tools for dining expenses, while those with fluctuating income see different cash flow visualisations than salaried customers. This contextual awareness extends to major life events, with the system recognising patterns indicating home purchases or travel planning and adjusting guidance accordingly.
This personalisation strategy has delivered impressive results: internal data reported at Money 20/20 Europe showed customers receiving personalised insights demonstrated 31% higher engagement rates and 23% higher retention compared to control groups. Perhaps most significantly, these customers reported substantially higher financial confidence scores, demonstrating personalisation's impact beyond traditional engagement metrics.
Media: The Financial Times's Content Relevance Strategy
The Financial Times faced the challenge of delivering personalised content experiences whilst maintaining editorial integrity and exposing readers to diverse perspectives. Their solution combines algorithmic recommendation with editorial curation in a system they call "Targeted Content."
This approach involves sophisticated analysis of reader behaviour to identify content affinities and topic interests, then using these insights to personalise various touchpoints including email newsletters, mobile alerts and website recommendations. Critically, the system balances personalisation with serendipitous discovery, ensuring readers maintain exposure to important coverage outside their demonstrated interests.
Implementation required developing a proprietary analytics framework that tracks engagement across multiple dimensions, including depth of reading (measured by scroll depth and time spent), content completion rates and subsequent actions (sharing, commenting or related content consumption).
The Financial Times reported that personalised content recommendations increased click-through rates by 30% and subscriber engagement by 14% compared to non-personalised alternatives. The initiative has proven particularly valuable for subscriber retention, with readers receiving personalised recommendations demonstrating substantially higher renewal rates according to data shared at the INMA World Congress.
Measurement and Optimisation
Defining Success Metrics
Establishing precise, meaningful metrics is essential for evaluating personalisation effectiveness and guiding ongoing optimisation. These metrics should span multiple dimensions, capturing both immediate performance indicators and longer-term business impact.
Engagement metrics serve as early indicators of personalisation relevance, revealing whether tailored experiences are resonating with recipients. Key engagement indicators include open rates, click-through rates, time spent with content and interaction depth (such as product views per session or article completion rates). While valuable, these metrics should be considered diagnostic tools rather than ultimate measures of success.
Conversion metrics provide more direct evidence of commercial impact, tracking how personalisation influences specific desired actions. Depending on your business model, these might include purchase completion rates, subscription signups, qualified lead generation or application submissions. When evaluating conversion impact, consider both immediate conversion lift and the cumulative effect across multiple touchpoints.
Customer value metrics offer the most comprehensive assessment of personalisation effectiveness, tracking how tailored experiences influence long-term value. Critical indicators include customer lifetime value, retention rates, share of wallet and advocacy behaviours such as referrals and social sharing. These metrics reveal whether personalisation is building sustainable relationships rather than merely optimising short-term transactions.
Financial metrics translate personalisation impact into business performance indicators, connecting customer experience improvements to revenue, profitability and operational efficiency. Key financial measures include revenue per customer, average order value, acquisition cost efficiency and support cost reduction. These metrics help justify continued investment in personalisation capabilities whilst identifying the most commercially impactful applications.
Continuous Improvement Approaches
Establishing systematic approaches to continuous improvement ensures personalisation effectiveness increases over time rather than stagnating after initial implementation. This involves both tactical optimisation of specific elements and strategic evolution of your overall approach.
Testing frameworks provide the foundation for tactical improvement, allowing you to systematically evaluate personalisation variants against control experiences. Traditional A/B testing works well for discrete elements like subject lines or call-to-action buttons, whilst more sophisticated multivariate approaches are required for complex personalisation systems with interdependent variables.
When designing tests, focus on learning velocity rather than just immediate performance lift. Prioritise tests that build understanding of fundamental customer preferences and behaviours rather than merely optimising execution details. For example, testing different personalisation approaches for new versus returning customers builds strategic knowledge more valuable than minor creative optimisations.
Feedback integration mechanisms complement quantitative testing by capturing qualitative insights directly from customers. These might include explicit feedback channels (such as surveys or preference centres), implicit signals (like content consumption patterns) and direct customer research through interviews or usability studies. The most effective organisations synthesise these diverse inputs into a comprehensive view of personalisation impact.
Learning documentation systems transform individual insights into institutional knowledge, ensuring discoveries from each optimisation cycle inform future strategy. Develop a structured approach to documenting not just what works but why it works, creating a growing library of personalisation principles specific to your customers and business context.
Future Horizons
Emerging Capabilities
The personalisation landscape continues evolving rapidly, with several emerging capabilities poised to transform current approaches. Understanding these developments helps organisations prepare for the next generation of customer experiences.
Contextual personalisation represents perhaps the most significant frontier, moving beyond historical preferences to incorporate real-time situational factors. This approach considers elements like location, weather conditions, current device, immediate intent signals and environmental context to deliver experiences uniquely relevant to the customer's circumstances at that precise moment.
For example, travel company Booking.com adjusts recommendations based not just on past booking patterns but current weather conditions at the user's location, upcoming holidays and even local events that might motivate travel planning. This contextual awareness creates significantly more relevant experiences than static preference-based approaches.
Multi-dimensional personalisation expands beyond content selection to personalise multiple experience elements simultaneously. Rather than simply recommending different products, this approach tailors messaging style, information density, visual presentation and interaction patterns to individual preferences. For instance, some customers respond better to data-rich presentations whilst others prefer emotionally resonant storytelling; multi-dimensional personalisation addresses these differences comprehensively.
Predictive personalisation moves from reactive to anticipatory experiences, using advanced analytics to forecast needs before customers explicitly express them. This capability enables brands to prepare relevant offers, content and services aligned with projected future needs rather than responding to established patterns. Early implementations include predicting category expansions in retail, identifying potential service issues before they occur and anticipating life stage transitions that alter product needs.
Ethical Considerations
As personalisation capabilities advance, ethical considerations become increasingly important. Organisations must navigate complex questions around data usage, algorithmic bias and consumer autonomy whilst building trust-based relationships.
Transparency represents the foundation of ethical personalisation, with customers understanding what data is collected, how it's used and what control they maintain. Leading organisations are moving beyond perfunctory privacy policies to develop clear, accessible explanations of their personalisation practices, including intuitive controls that allow customers to adjust parameters or opt out entirely if desired.
Algorithmic fairness presents another critical consideration, ensuring personalisation systems don't inadvertently perpetuate biases or create inequitable experiences. This requires regular auditing of algorithms for potential bias, particularly regarding price sensitivity, offer allocation and content selection. Organisations should establish clear guidelines for when differential treatment represents valuable personalisation versus problematic discrimination.
Value exchange clarity ensures customers perceive fair compensation for the data they provide. This involves articulating the concrete benefits customers receive through personalisation rather than merely collecting data because technology enables it. When customers understand the connection between shared information and enhanced experiences, they typically demonstrate greater willingness to participate in personalisation programmes.
Conclusion
Personalisation at scale represents not merely a technical capability but a fundamental reimagining of the relationship between brands and customers. By transforming impersonal transactions into contextually relevant experiences, organisations can forge deeper connections that create lasting competitive advantage.
The journey toward truly effective personalisation combines sophisticated data capabilities with human creativity and ethical stewardship. Organisations that strike this balance, using technology to enhance rather than replace human judgment, will deliver experiences that resonate on both rational and emotional levels.
As you develop your personalisation strategy, remember that the ultimate goal extends beyond immediate performance metrics to building sustained, trust-based relationships with customers. By focusing on delivering genuine value through relevant experiences, you create the foundation for long-term loyalty that transcends individual transactions or campaigns.
The most successful practitioners view personalisation not as a static destination but as an ongoing commitment to increasingly meaningful customer relationships. This perspective transforms personalisation from merely a marketing tactic into a core business philosophy that shapes every aspect of customer engagement.
Frequently Asked Questions
What is the difference between segmentation and true personalisation at scale?
Segmentation divides customers into defined groups with similar characteristics, whereas true personalisation adapts experiences based on individual behaviours and preferences. While segmentation might create dozen of customer groups receiving different experiences, personalisation at scale potentially creates millions of unique experience variations. The key difference lies in granularity: segmentation applies the same treatment to everyone within a segment, whilst personalisation considers the unique attributes of each individual customer.
How can organisations balance personalisation with privacy concerns?
Successful organisations approach this balance through three key practices: transparent data policies that clearly explain what information is collected and how it's used; meaningful value exchange that ensures customers receive tangible benefits from sharing data; and progressive permission models that allow customers to increase data sharing gradually as they experience the benefits. Additionally, adopting privacy-by-design principles ensures protection is built into personalisation systems rather than added as an afterthought.
What organisational structures best support personalisation at scale?
The most effective structures combine centralised strategy with distributed execution capabilities. This typically involves a central team responsible for data architecture, technology platforms and measurement frameworks, working alongside embedded specialists within business units who apply these capabilities to specific customer journeys. This hybrid approach ensures consistency in approach whilst maintaining the contextual understanding required for relevant implementation.
How should we measure the ROI of personalisation investments?
Comprehensive ROI measurement combines multiple metrics across timeframes: short-term performance indicators like conversion rate improvements and average order value increases; medium-term metrics such as customer retention rates and purchase frequency; and long-term measures including customer lifetime value and advocacy behaviours. The most accurate assessments also include operational efficiency gains from automated decisioning and reduced marketing waste. When calculating investment returns, consider both technology costs and the organisational capabilities required for effective execution.
How will emerging technologies like artificial intelligence transform personalisation practices in the future?
AI will enable more sophisticated approaches including predictive personalisation that anticipates needs before explicit expression; contextual awareness that incorporates real-time situational factors; multivariate optimisation across content, timing, channel and messaging simultaneously; and dynamic journey orchestration that adapts entire customer experiences rather than isolated touchpoints. These capabilities will shift personalisation from primarily reactive to increasingly anticipatory, with systems that learn continuously from each interaction to improve future experiences.
References and Further Reading
To learn more about the case studies mentioned in this article, consider researching:
- "ASOS personalisation strategy eConsultancy retail case study" - eConsultancy's detailed analysis examines ASOS's implementation approach and provides specific metrics on conversion improvements and revenue impact.
- "Monzo app personalisation Money 20/20 Europe presentation" - Money 20/20 conference proceedings include Monzo's technical approach to financial personalisation and specific engagement metrics.
- "Financial Times content personalisation strategy INMA presentation" - The International News Media Association's documentation of the FT's approach to balancing algorithmic recommendation with editorial judgment.
- "Booking.com contextual personalisation case study Harvard Business Review" - HBR's analysis details Booking.com's implementation of real-time contextual factors in their recommendation engine.
- "McKinsey personalisation ROI retail banking report 2021" - McKinsey's comprehensive study provides industry benchmarks for personalisation impact across multiple sectors.